Deep Learning for THz Drones with Flying Intelligent Surfaces
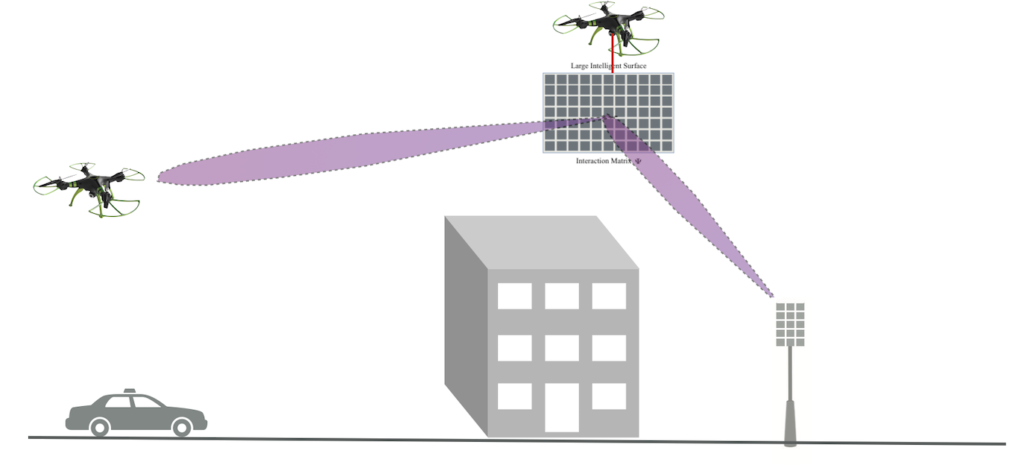
We propose incorporating large intelligent surfaces to the drones at THz networks and leveraging them for proactive handoff and beam prediction for the highly-mobile and reliability-sensitive applications
Key ideas
- Leverage flying RIS to enhance mmWave/THz network reliability and mobility support
- Using machine learning to proactively predict RIS reflection beams
- Proactively predicting drone hand-off decision between BSs/RISs
Applications
- Leveraging RIS (deployed for example at drones) to enhance the network reliability/latency
- Supporting drone communications with the aide of flying RISs
More information about this research direction
Paper: Nof Abuzainab, Muhammad Alrabeiah, Ahmed Alkhateeb, and Yalin E. Sagduyu, “Deep Learning for THz Drones with Flying Intelligent Surfaces: Beam and Handoff Prediction,” arXiv preprint arXiv:2102.11222 (2021).
Abstract: We consider the problem of proactive handoff and beam selection in Terahertz (THz) drone communication networks assisted with reconfigurable intelligent surfaces (RIS). Drones have emerged as critical assets for next-generation wireless networks to provide seamless connectivity and extend the coverage, and can largely benefit from operating in the THz band to achieve high data rates (such as considered for 6G). However, THz communications are highly susceptible to channel impairments and blockage effects that become extra challenging when accounting for drone mobility. RISs offer flexibility to extend coverage by adapting to channel dynamics. To integrate RISs into THz drone communications, we propose a novel deep learning solution based on a recurrent neural network, namely the Gated Recurrent Unit (GRU), that proactively predicts the serving base station/RIS and the serving beam for each drone based on the prior observations of drone location/beam trajectories. This solution has the potential to extend the coverage of drones and enhance the reliability of next-generation wireless communications. Predicting future beams based on the drone beam/position trajectory significantly reduces the beam training overhead and its associated latency, and thus emerges as a viable solution to serve time-critical applications. Numerical results based on realistic 3D ray-tracing simulations show that the proposed deep learning solution is promising for future RIS-assisted THz networks by achieving near-optimal proactive hand-off performance and more than 90% accuracy for beam prediction.
@misc{Abuzainab2021,
title={Deep Learning for THz Drones with Flying Intelligent Surfaces: Beam and Handoff Prediction},
author={ Abuzainab, Nof and Alrabeiah, Muhammad and Alkhateeb, Ahmed and Sagduyu, Yalin E.},
year={2021},
eprint={2102.11222},
archivePrefix={arXiv},
primaryClass={cs.IT}}
To reproduce the results in this paper:
Simulation codes (based on DeepMIMO v1):
Coming soon
Example: Steps to generate the results in this figure
- Coming soon.